This week's blog post is contributed by guest writer, Connie Vaughn, Director of Data Science for NaviStone. Connie has worked in data science and analytic marketing for over twenty years for a variety of agencies and client companies. Her favorite parts of data science are getting creative with data elements, using mathematics to simplify business problems, and creating data products that directly impact the business. She has degrees in math, psychology, business, and journalism, as well as a certificate in teaching English to speakers of other languages. She is getting ready to foster-to-adopt a teenager and hoping to give her first TEDx talk in the near future.
Making Direct Mail...Direct Again
I’ve been around long enough to remember the pre-modeling days of retail, when we used “RFM” to score and rank customers—Recency, Frequency, Monetary. This was essentially a three-dimensional cube for sorting and segmenting customers, based solely on their past purchase behavior aggregated from retail order files. Predictive modeling and CRM systems came along and used more variables and maybe a few demographics, but it was still the same principle: “Past behavior is the best predictor of future behavior.” Or so we said to ourselves and our clients.
But is it always?
Customer models based on past purchases explained 1-3% of the subsequent purchase behavior. Human behavior is notoriously challenging to predict, and probably always will be—but these results were dismal. We used them, because anything better than chance still helped us make money. But the noise in our targeting was high—and our direct mail was often “junk mail” to the consumer.
We had even bigger problems with prospecting for new customers. We had no past purchase behavior for non- (or not-yet) customers. We relied on lists of names from magazines and data compilers, and in the best-case scenario, co-op data that included our competitors. It was common to have prospecting response rates less than 1%, and to take well over a year to recoup the costs to acquire a single new customer. And we generated a lot of junk mail in the process.
Then, the Internet happened. The retail world moved away from seemingly expensive mail, and into seemingly cheap email, digital display, search optimization, and retargeting.
Modeling didn’t immediately follow these new channels. Some channels, such as email, were cheap enough to blanket the market. There was little down side. I’ve personally done studies of opt outs, and even irritated customers delete emails far more than they unsubscribe.
In other cases, resellers developed simple to complex audience segmentations. Much retargeting was based on a single product view and couldn’t even tell whether you actually purchased the product or not. On the other extreme, Google and Facebook have become unwittingly notorious examples of some of the socially-charged characteristics an advertiser could select on complex, poorly-monitored platforms.
Meanwhile, customers learned to ignore online ads and emails, and returns on these “cheap” channels dropped precipitously.
Enter Web-powered Direct Mail: A solution that has been developed in which online browsing behavior can be linked—in a blind, privacy-compliant manner— to a customer’s name and mailing address. With mailboxes so much less cluttered nowadays, a simple offline mail piece to a website visitor stands out, and can nudge the customer to a return visit and a purchase. Suddenly the returns on investment from direct mail actually outperform their online advertising counterparts.
Several players in the marketplace offer this web-to-mail service. But a true differentiator to making it successful is applying data science techniques to visitors’ browsing behavior, by capturing every click and action of every visitor in a big data repository. With all this data, not only can a visitor be identified for direct mail—but advertisers can learn which visitors to mail profitably, and which to ignore.
Think about it. Browsing behavior isn’t someone’s past buying behavior—it’s a real-time indication of product interest and engagement. Browsing sends a live signal of intent to purchase. Patterns of browsing—such as repeat visits, types of products viewed, and time spent on site—distinguish true interest from “window shopping.” They tell us the story of a visitor’s location and timing on their path to purchase.
With the rich data from browsing combined with advanced pattern-recognition methodologies, advertisers can finally use data, technology, and modeling to make direct mail personal. Just like we used to say in CRM!
The new browsing behavior models explain 8-12% of a visitor’s subsequent purchase behavior. Humans are still more capricious than not, but the signal that allows us to accurately target has increased tenfold! It is as if, in the heyday of print catalogs, we had been able to sit on the customer’s shoulder and note which products they browsed and searched, and the page corners they folded down to return to.
Mailers can be more effective in generating profitable prospecting, housefile, and reactivation campaigns. Instead of relying on vague interest data or past purchases, they now know who is browsing their site and how serious they are, before they have (re-)identified themselves via a purchase or sign-up. Creative and product personalization are also possible.
So far there has been no indication that customers find the Web-powered mail to be intrusive or “creepy.” We’ve all become used to ads following us around the Web, and mail is considerably less intrusive than that.
Mail is also less likely to be ignored than an online ad or email. One of the surprising finds made recently is the long life that a postcard program has, once it’s in a customer’s hands. It was once assumed that the response window on this first-class mailer would be two to thirty days. But people hang onto the physical postcard for sixty days, ninety days, and even beyond. They sometimes hang onto them and respond even after an offer period has ended and they don’t get the special price! Thus, mail serves as a branding vehicle as much as a response vehicle.
Considered purchases with high average order values benefit from this approach, in particular. Website visitors to these sites are on a journey of information-gathering and decision-making. This includes eCommerce sites as well as sales lead generation for purchases transacted offline (e.g., automobiles, mortgages). Being touched by a mail piece that they can hang onto, perhaps at several points along their buying journey, generates some of the highest returns we have achieved.
Another recent finding is that Web-powered direct mail incites visitors to return to the branded Website to complete their purchase, rather than making an Amazon or eBay marketplace transaction for the same product. This benefits advertisers who do not have to pay the reseller, and reinforces the brand’s relationship with the customer. With Amazon accounting for upwards of 43% of all online sales, a direct branding and customer relationship vehicle becomes ever more important for retailers.
Personally speaking, right now is an exciting time to be a data scientist. The innovation in using browsing data to drive offline marketing fuses the benefits of current technology, data, and modeling. We are able to deliver twenty-first century branded, relevant, personalized, and high-response direct mail which is now anything but “junk.”
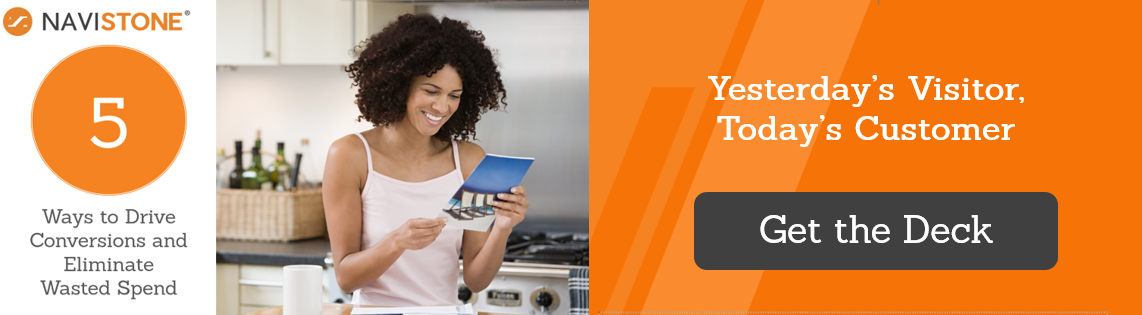
Comments